We have a new essay published on Deloitte Insights, Why hasn’t AI delivered on its promise?,1Evans-Greenwood, Peter, Amina Crooks, and Kellie Nuttall. “Why Hasn’t AI Delivered on It’s Promise?” Deloitte Insights, December 16, 2022. https://www2.deloitte.com/us/en/insights/topics/emerging-technologies/ai-adoption-challenges.html. a collaboration between the Centre for the Edge and the AI Institute. This time we’re smashing together the ideas from The real landscape of technology-enabled opportunity2Evans-Greenwood, Peter. “The Real Landscape of Technology-Enabled Opportunity.” Deloitte Insights, February 28, 2022. https://www2.deloitte.com/us/en/insights/topics/innovation/technology-opportunity-landscape.html. and Reconstructing jobs3Evans-Greenwood, Peter, Alan Marshall, and Matthew Ambrose. “Reconstructing Jobs: Creating Good Jobs in the Age of Artificial Intelligence.” Deloitte Insights, July 18, 2018. https://www2.deloitte.com/insights/us/en/focus/technology-and-the-future-of-work/creating-good-jobs-age-of-artificial-intelligence.html to see if they can help us understand why, despite recent advances in AI, adoption seems to be lacking.
AI, it’s been predicted, will disrupt pretty much everything. With the recent releases of Midjourney and Stable Diffusion we’re in the midst of another round of predictions that these new tools will disenfranchise humans, pushing us out of jobs etc. Back in 2016 Hinton predicted that it would soon be pointless to train human radiographers,4Smith, Gary, and Jeffrey Funk. “AI Has a Long Way to Go before Doctors Can Trust It with Your Life.” Quartz, June 5, 2021. https://qz.com/2016153/ai-promised-to-revolutionize-radiology-but-so-far-its-failing/. now we have predictions that the entire graphic arts community will be out of work in short order. (Predictions which are likely to be wrong.)
Despite all this alarm, AI penetration into business is quite low. There’s a lot of investment, but most (possibly something like 85%, according to Gartner) projects fail.5Gartner predicted in 2019 that by 2022 some 85% of AI projects would fail. See: Andrew White, “Our top data and analytics predictions for 2019,” blog, Gartner, January 3, 2019. It’s difficult to support this prediction with data, likely as firms are often reluctant to report on failures. Figures between 60 and 80% are often quoted, which aligns with estimates from practitioner experience. This is roughly double the usual failure rate for IT projects. What seem like good ideas and compelling prototypes aren’t making the transition into value-creating solutions.
The main idea in The real landscape of technology-enabled opportunity is that most (if not all) disruptive technologies are the result of incremental innovation, the slow development of otherwise banal technologies that somehow tip into world-changing innovations. It’s rare for an innovator having a lightbulb moment in the shower to lead to world changing solution. Consider, for example, the steam engine. If we were to pin the emergence of the modern steam engine (triggering the Industrial Revolution) to a particular technology, then we might as well pick the development of machine tools. Humans had been experimenting with steam engines for a long time, but it was only with the development of precision machine tools6See The Prefectionists for a popular introduction to the history of precision and machine tools. Winchester, Simon. The Perfectionists: How Precision Engineers Created the Modern World. First Harper Perennial edition. New York: Harper Perennial, 2019. (machines that make machines) that it was possible to make the parts for a modern steam engine.
If we look at the emergence of modern AI solutions—such as machine translation—then we can see a similar dynamic in action. Machine translation is an old idea: it was first mentioned in the Fifties and the theory for modern machine translation was established in the Eighties. The technology emerged into the popular consciousness in the 2000s not due to the theoretical developments, but to the release of bilingual texts and access to cheap compute.
If we’re to ascribe the emergence of the last wave of AI solutions to anything, then the best option is the shift from a world with some digital tools to one saturated by digital technology, which occurred into the early to mid 2000s. Things that were expensive, like accessing digital bilingual texts, became cheap or even free, unleashing a wave of innovation. Things that were technically possible but economically challenging suddenly became easy. Our mistake was to assume that this was due to developments in technology, rather than developments in the things that those technologies relied on.
This is where Reconstructing jobs comes in. Back in 2019 we pointed out if we want to make the most of AI, then we need to think about work differently. We assume that AI is a task-performing technology, but it might better be conceived as a technology to create automated behaviours—sense-respond rather than do. We need to think about work differently if we want to make the most of the technology. If we develop this approach then we end up with Figure 2 from Why hasn’t AI delivered on it’s promise?
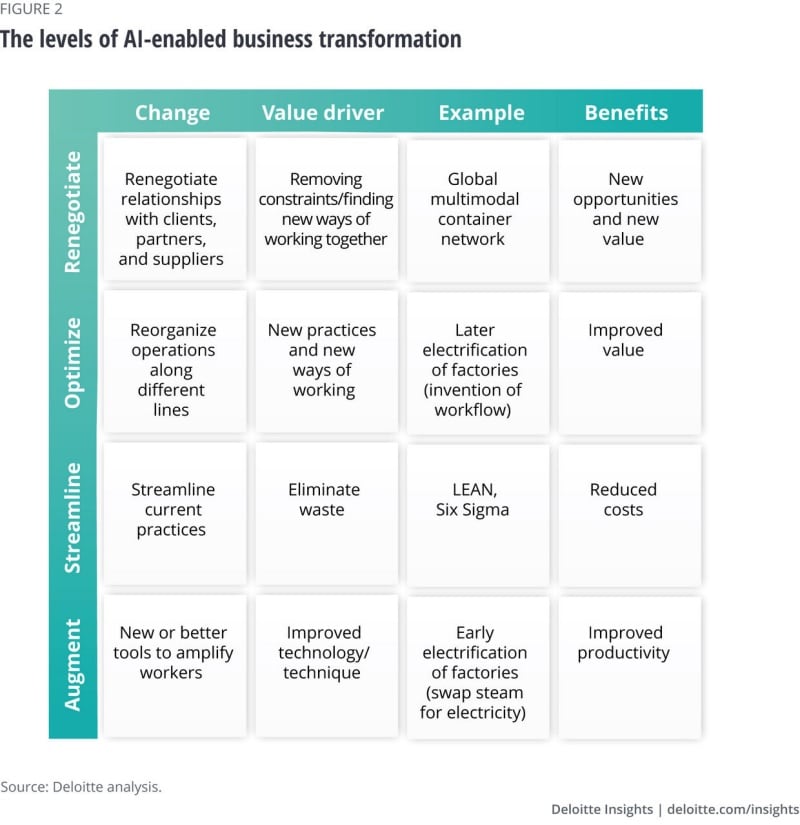
Our default approach to a new technology is to consider it a tool to do a task. AI is a tool for creating perfect predictions, so we need to find predictions to make perfect. There is some utility to this approach, but much of the opportunity AI can provide lies elsewhere, in problems where we change our approach to work, and where AI is only part of the solution (rather than the whole solution). The global multi-modal container network is a great example, where a good idea and otherwise banal technologies was brought together to reorganise mode transfers—from truck-to-ship, ship-to-truck—slashing transport costs and ushering in the current phase of globalisation.
AI has a lot of potential, we just need to look outside the streetlight of disruptive innovation.
You can find Why hasn’t AI delivered on its promise? on Deloitte Insights.
Endnotes
- 1Evans-Greenwood, Peter, Amina Crooks, and Kellie Nuttall. “Why Hasn’t AI Delivered on It’s Promise?” Deloitte Insights, December 16, 2022. https://www2.deloitte.com/us/en/insights/topics/emerging-technologies/ai-adoption-challenges.html.
- 2Evans-Greenwood, Peter. “The Real Landscape of Technology-Enabled Opportunity.” Deloitte Insights, February 28, 2022. https://www2.deloitte.com/us/en/insights/topics/innovation/technology-opportunity-landscape.html.
- 3Evans-Greenwood, Peter, Alan Marshall, and Matthew Ambrose. “Reconstructing Jobs: Creating Good Jobs in the Age of Artificial Intelligence.” Deloitte Insights, July 18, 2018. https://www2.deloitte.com/insights/us/en/focus/technology-and-the-future-of-work/creating-good-jobs-age-of-artificial-intelligence.html
- 4Smith, Gary, and Jeffrey Funk. “AI Has a Long Way to Go before Doctors Can Trust It with Your Life.” Quartz, June 5, 2021. https://qz.com/2016153/ai-promised-to-revolutionize-radiology-but-so-far-its-failing/.
- 5Gartner predicted in 2019 that by 2022 some 85% of AI projects would fail. See: Andrew White, “Our top data and analytics predictions for 2019,” blog, Gartner, January 3, 2019. It’s difficult to support this prediction with data, likely as firms are often reluctant to report on failures. Figures between 60 and 80% are often quoted, which aligns with estimates from practitioner experience. This is roughly double the usual failure rate for IT projects.
- 6See The Prefectionists for a popular introduction to the history of precision and machine tools. Winchester, Simon. The Perfectionists: How Precision Engineers Created the Modern World. First Harper Perennial edition. New York: Harper Perennial, 2019.